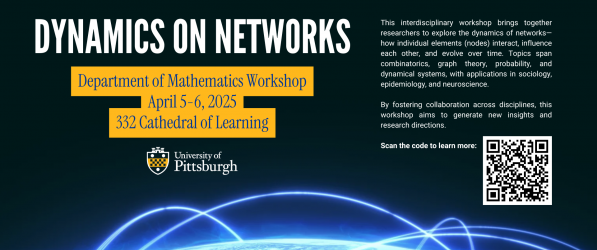
This workshop will bring together researchers from different disciplines to exchange ideas stemming from diverse perspectives on dynamics on networks. The focus of the workshop can be summarized in terms of a central construction: Consider a collection of elements, individuals – more generally, nodes – that can each take on one of several states at any given time. Suppose that nodes interact in a way that (a) depends on their states and their connections, and (b) may cause their states to change. In this setting, a wide range of questions arise that span topics in combinatorics, network and graph theory, probability theory, and dynamical systems, with applications in fields such as sociology, epidemiology, and neuroscience. These questions include issues of global architecture, such as how the eventual pattern of states across an interacting network of nodes depends on the overall network structure; issues of local initialization, such as what properties of local subsets of nodes can allow them to strongly influence the rest of the network; and issues of dynamics, relating to how the set of available states and the rules for transitioning between them affect pattern formation in the network. By promoting interaction of researchers who have disparate perspectives and expertise relevant to aspects of this general problem class, this workshop will fertilize new collaborations and progress on questions of interest to a range of communities.
Location and Address
332 Cathedral of Learning
Speaker Information
Hamed Amini (University of Florida)
Abstract: Dynamics of Cascading Losses in Stochastic Networks
We study the dynamics of default cascades and loss propagation in large stochastic networks. We derive limit theorems for contagion size and assess network resilience in relation to network heterogeneity and structure. Our findings also offer insights into optimal intervention strategies for mitigating systemic risk in large complex networks.
Dani Bassett (University of Pennsylvania)
Abstract: Control Strategies for Dynamic Network Systems
Neural systems in general—and the human brain in particular—are organized as networks of interconnected components. Across a range of spatial scales from single cells to macroscopic areas, biological neural networks are neither perfectly ordered nor perfectly random. Their heterogeneous organization supports complex activity dynamics while simultaneously constraining such dynamics. How does the network constraint affect the cost of neural activity flow? In this talk, I will use the formalism of network control theory to define a notion of network economy: the idea that a biological neural network’s organization partially determines the cost of reaching a neural state, maintaining a neural state, and transitioning between neural states. I will demonstrate how the principle of network economy can guide our study of neural system function in health and disease and inform our understanding of neural computation.
Igor Belykh (Georgia State University)
Abstract: Cyclops states in oscillator networks with higher-mode coupling
Oscillator networks, including neuronal ensembles, can exhibit multiple cooperative rhythms such as chimera and cluster states. However, understanding which rhythm prevails remains challenging. In this talk, we address this fundamental question in the context of Kuramoto-Sakaguchi networks of rotators with higher-order Fourier modes in the coupling. We show that three-cluster splay states with two distinct, coherent clusters and a solitary oscillator are the prevalent rhythms in networks with an odd number of units. We denote such tripod patterns cyclops states with the solitary oscillator reminiscent of the Cyclops' eye. As their mythological counterparts, the cyclops states are giants that dominate the system's phase space in weakly repulsive networks with first-order coupling. Astonishingly, adding the second or third harmonics to the Kuramoto coupling function makes the Cyclops states global attractors practically across the full range of coupling's repulsion. Beyond the Kuramoto oscillators, we analytically demonstrate that cyclops states are robustly present in networks of canonical theta-neurons with adaptive coupling. More generally, our results suggest clues for finding dominant rhythms in physical and biological networks.
Richard Betzel (University of Minnesota)
Abstract: Spreading models on multi-scale connectomes
Connectomes are wiring diagrams of nervous systems. The organization of a connectome's elements -- its nodes and edges -- constrains brainwide signaling/communication patterns. Consequently, the connectome plays an important role in relaying information about an organism's environment from sensory neurons to other parts of the brain, where that information can contribute to decision-making, navigation, action planning, etc. Here, we use an idealized and abstract model to simulate sensory cascades in human and Drosophila connectomes. The results of these simulations allow us to investigate and compare the spatiotemporal trajectories associated with single sensory modalities (in isolation), as well as synergistic and competitive multi-sensory cascades, identifying potential integrative convergence sites in the insect and human brain.
Leah Keating (University of California, Los Angeles)
Abstract: An Approximate-Master-Equation Formulation of the Watts Threshold Model on Hypergraphs
In traditional models of behavioral or opinion dynamics on social networks, researchers suppose that all interactions occur between pairs of individuals. However, in reality, social interactions also occur in groups of three or more individuals. A common way to incorporate such polyadic interactions is to study dynamical processes on hypergraphs. In a hypergraph, interactions can occur between any number of the individuals in a network. The Watts threshold model (WTM) is a well-known model of a simplistic social spreading process. Very recently, Chen et al. extended the WTM from dyadic networks (i.e., graphs) to polyadic networks (i.e., hypergraphs). In this talk, I will explain how we extended their discrete-time model to continuous time using approximate master equations (AMEs). By using AMEs, we are able to model the system with very high accuracy. We then reduce the high-dimensional AME system to a system of three coupled differential equations without any detectable loss of accuracy. This much lower-dimensional system is more computationally efficient to solve numerically and is also easier to interpret. We linearize the reduced AME system and calculate a cascade condition, which allows us to determine when a large spreading event occurs. We then apply our model to a social contact network of a French primary school and to a hypergraph of computer-science coauthorships. We find that the AME system is accurate in modelling the polyadic WTM on these empirical networks.
Laurent Hébert-Dufresne (University of Vermont)
Abstract: How things spread when their fitness vary: Complexity of anisotropic spreading models
Models of how things spread often assume that transmission mechanisms are fixed over space and time. However, the transmission of infectious diseases can depend on local human behaviour or quality of the surrounding infrastructure. Likewise, social contagions like the spread of ideas, beliefs, and innovations depend on local norms or culture, and they can lose or gain in momentum as they spread. Diseases mutate and find subpopulations where they can thrive, just as ideas can get reinforced, beliefs strengthened, and products refined. We study the impacts of these mechanisms in spreading and cascade dynamics. Using different modelling tools, we find that complexity and criticality are the norm: Superlinear relationships between exposure and transmission can emerge from linear interactions and power-law distributions can be observed away from any critical point.
Nynke Niezink (Carnegie Mellon University)
Abstract: Statistical models for dynamics on dynamic networks
Social networks play a major role in shaping individual decision-making. Most social networks are not static; like individual behavior, they evolve continuously over time. To understand the origins and impacts of people's network position, it is important to comprehend the mechanisms governing network change and the effect of networks on individual behavior, such as through social influence. The stochastic actor-oriented model is a statistical model that enables the study of network dynamics using longitudinal data. In this talk, I will introduce an extension of this model to analyze the co-evolution of social networks and continuous behavior variables (such as performance or health-related outcomes). The framework models attribute evolution through a stochastic differential equation and network evolution using a Markov chain model. I will illustrate the model through a study of the role of peers in the development of stress among students in higher education.
Sevak Mkrtchyan (University of Rochester)
Abstract: The limit shape of the leaky Abelian sandpile model with multiple layers
The leaky abelian sandpile model (Leaky-ASM) is a growth model in which n grains of sand start at the origin in the square lattice and diffuse according to a toppling rule. A site can topple if its amount of sand is above a threshold. In each topple a site sends some sand through the network and leaks a certain portion of its sand. This is a dissipative generalization of the Abelian Sandpile Model, in which no sand leaks.
The sandpile eventually stabilizes, and one of the important questions about the model is the study of the final stable configuration. We will discuss how, by connecting the model to a certain killed random walk on the underlying graph, an explicit limit shape can be computed for the region visited by the sandpile when it stabilizes.
Originally the model was studied for nearest neighbor interactions on the square lattice in dimension 2. We will discuss a vast generalization where the dimension can be anything, each site can hold several different stacks of sand, one for each of a certain given number of different layers or colors, and lastly, when a stack of one color at a site topples, it can send sand not only to its nearest neighbors in equal amounts, but to all possible locations and colors, according to a fixed but arbitrary mass distribution.
Ani Sridhar (Massachusetts Institute of Technology)
Abstract: New Perspectives on Learning Structure from Dynamics
Suppose that a continuous-time, stochastic diffusion (e.g., an epidemic or cascade) spreads on an unknown graph. We only observe the time at which the diffusion reaches each vertex, i.e., the set of "infection times". What can be learned about the unknown graph from the infection times? While there is far too little information to learn individual edges in the graph from a single diffusion, we show that certain high-level properties -- such as the number of vertices of sufficiently high degree -- can surprisingly be determined with certainty. To achieve this goal, we develop a suite of algorithms that can efficiently estimate vertices of degree asymptotically larger than $\sqrt{n}$ from infection times, for a natural and general class of graphs with $n$ vertices. To complement these results, we show that our algorithms are information-theoretically optimal: there exist graphs for which it is impossible to tell whether vertices of degree larger than $n^{1/2 - \epsilon}$ exist from the vertices' infection times, for any $\epsilon > 0$. Finally, we discuss the broader implications of our ideas for change-point detection in non-stationary point processes. This talk is based on joint work with Anna Brandenberger and Elchanan Mossel.
Filippo Radicchi (Indiana University)
Abstract: Modeling resource consumption via minimum-cost percolation
In this talk, I introduce a dynamic percolation model designed to describe resource consumption and depletion in networks: the minimum-cost percolation (MCP) model. In the model, rational agents progressively consume resources allocated on the edges of a network along demanded minimum-cost paths. As a result, the network undergoes a transition between a percolating phase where it can properly serve demand to a non-percolating phase where demand can no longer be supplied. First, I investigate the MCP transition on Erd\H{o}s–Rényi (ER) and uncorrelated scale-free (SF) networks. On ER graphs, the nature of the phase transition changes depending on the budget available to the agents. Finite budget leads to a standard continuous percolation phase transition; the transition becomes instead weakly discontinuous if budget is unbounded. On SF graphs, the power-law degree distribution is responsible for an anomalous phase transition only if paths of length one can be requested; for larger budget values instead, one recovers the same behavior as in ER graphs, displaying a continuous transition for finite budget and a weakly discontinuous transition in the infinite-budget case. Second, I apply the MCP model to a weighted, directed, temporal, multi-layer network representation of the air transportation system that can be generated using real schedules of commercial flights operated by US carriers. I study how cooperation among different carriers could improve the ability of the overall air transportation system in serving the demand of passengers, finding that unrestricted cooperation could lead to a 30% efficiency increase compared to the non-cooperative scenario. Cooperation would require major airlines to share a significant portion of their market, but it would allow also for an increased robustness of the system against perturbations causing flight cancellations. My findings underscore some key benefits that could emerge by simply promoting code-share arrangements among US airlines without altering their current cost of operation.
For further information, please contact organizers:
- Jonathan Rubin (jonrubin@pitt.edu)
- Sabrina Streipert (streipert@pitt.edu)